Analysis
Unmasking Deepfakes: Exploring Amped Authenticate’s Advanced Detection Features
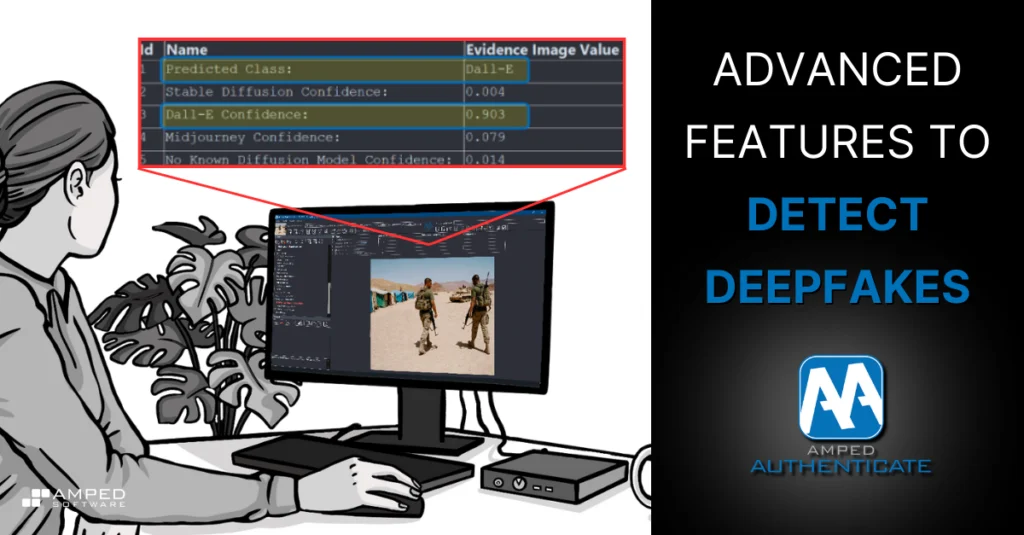
ADVERTORIAL: By Massimo Iuliani, Forensic Analyst at Amped Software
When deepfakes first emerged, they exhibited evident visual inconsistencies that could typically be detected through simple visual inspection. However, in 2021 a scientific research highlighted that people could not visually distinguish between natural and synthetic images anymore!
In the last three years, with the advent of diffusion models, there has been a noticeable improvement in the tools for the synthetic generation of images. Below, Amped Software presents some images. It is definitely challenging to visually determine if they are natural or synthetically generated.
Before moving on, it is important to clarify that deepfakes can be generated by different technologies. For instance, they can be created through Generative Adversarial Networks (GANs) or text-to-image models (also known as “diffusion models”, such as Dall-E, Midjourney, Stable Diffusion, Flux). You can find a very quick introduction to the differences between them on the Amped Software blog at this link. However, diffusion models are gaining prominence due to their ability to convert textual prompts into photorealistic images.
A first technical approach to detect this kind of content is the analysis of metadata. Indeed, tools for synthetic image generation may leave traces in the image container by storing some specific metadata.
For instance, a synthetically generated image’s metadata may reveal the prompt used for the text-to-image process or some AI-related tag.
Below, Amped Software presents a subset of the metadata extracted from an image generated through diffusion models.
The value “AI generated image” is clearly visible in the “ActionsDescription” field, which is very self-explanatory.
However, metadata analysis has prominent limits and can be hardly considered a definitive solution.
First, some AI-images are generated in .png or other formats with no relevant metadata. Below, you can see the metadata extracted from the first image shown above (depicting two soldiers in the desert in front of a refugee camp and a tank).
It can be observed that the image is described with less than 10 very general metadata fields. It is difficult to determine the origin of this image from these field values.
Second, even when some AI-related metadata are attached to the image during the generation process, they are generally removed in the next steps of the image life cycle. The most common situation is when the image is uploaded to a social network: most of the metadata is stripped, thus making their analysis useless for detecting deepfakes.
Last but not least, metadata can be manually modified by a user. This process, although technical, is feasible by a skilled user, thus limiting the reliability of metadata-based analysis in various contexts.
Luckily, recent research has highlighted that diffusion models leave some traces in the generated images. Among them, one of the latest papers highlighted that diffusion model traces can be detected through the so-called CLIP (Contrastive Language-Image Pre-training) features from the image [1]. This work showed excellent performance both in the scientific paper and Amped Software’s internal validation. That’s why Amped Software decided to equip Amped Authenticate with this specific deep-fake detection filter.
In this first release, Amped Software trained the system with a variety of real images and pictures from the most common text-to-image methods: Midjourney, Stable Diffusion, and Dall-E. When you process an image with the filter, you’ll get a tabular output showing the confidence score assigned by the classifier to each class. The scores sum to 1, and for user convenience, the class with the highest score is always reported in the “Predicted Class” row.
Let’s see the output of the tool on the same PNG image with missing metadata:
In this case, the filter shows that the image is strongly compatible with a synthetic generation process. More specifically, the image statistics are highly compatible with Dall-E (confidence 0.903).
As shown in the example above, the filter also provides a score for the “No Known Diffusion Model” class. An image with a high score in this class is compatible (according to the detector) with other synthetic generation methods, as any other unpredicted image life-cycles. In this case, however, the filter is only confident that the image is not compatible with one of the trained diffusion models.
Note that the detection method is based on machine learning and the classifier can get it wrong for several reasons:
– There are dozens of generation methods. The filter can get confused when analyzing a synthetic image generated by an unknown model. An example is that an image generated through Fooocus can be detected as a Dall-E image. However, in this case, the filter provides at least a clue that the image is synthetically generated.
– Generative models are frequently updated. This means that the filter can miss an image generated with a new, previously unseen version of a known model.
– Several generative models allow a deep-setting customization. It is unfeasible to predict how all available parameters can affect the traces on the generated image.
– The filter cannot be trained with all possible image life cycles. This means that its behavior cannot be completely predicted when the analyzed image has been subjected to a very unusual processing history.
As a consequence, a high confidence score does not guarantee that the predicted class is correct.
On the other hand, however, the filter detects traces that are generally robust to compression. This means that the filter has chances to work effectively even when the image is exchanged through a social network. For instance, if we exchange the previous image example (depicting two soldiers) through WhatsApp, the filter still predicts Dall-E with a high confidence (over 0.9).
In the end, Amped Authenticate’s Diffusion Model Deepfake filter is a valuable tool for countering deepfakes, especially in common situations where metadata is unavailable or unreliable.
[1] Cozzolino, D., Poggi, G., Corvi, R., Nießner, M., & Verdoliva, L. (2024). Raising the Bar of AI-generated Image Detection with CLIP. In Proceedings of the IEEE/CVF Conference on Computer Vision and Pattern Recognition (pp. 4356-4366).
Category: AdvertorialTechnology
Advertisement
Job of the week
Mersey Tunnels Police Constable (Transferee Only)
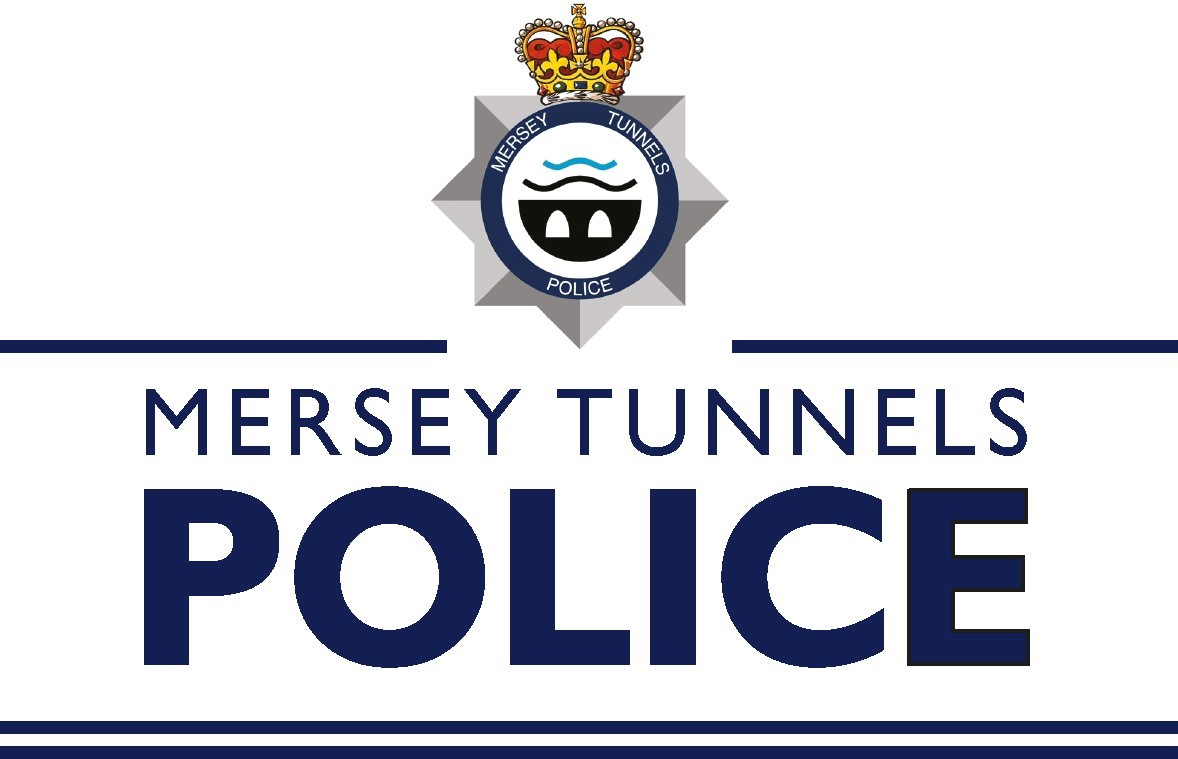
- MERSEY TUNNELS POLICE
- Mersey Tunnels Police Offices
- £38,119.21- £42,44.91
The Liverpool City Region is world-famous for its history, culture and creativity. A place of firsts, it is the birthplace of modern railways, public health and international trade. Now, our economy is being transformed once again, changing the world through world-leading innovation in health and life sciences, digital and creative, and advanced manufacturing - growing an economy already worth £35 billion a year. The Combined Authority plays a central role in catalysing this innovation-led economy. We are seizing the unique opportunity to use our devolved powers and funding to create wealth and prosperity for all our 1.6 million residents and the whole UK - investing in our economy, people, place, transport and digital infrastructure. Our vision is for the Liverpool City Region to be: The best place to grow up, grow a family, and grow a business - where no-one is left behind.
Read more